Machine learning and fractal geometry
MSc thesis, defended at the University of Amsterdam, supervised by Pieter Adriaans- 30 Mar 2010
My Master’s thesis was something of an exercise in lateral thinking. I had recently read James Gleick’s Chaos, and had become a little obsessed with things like fractals and chaos theory. Since I was studying AI, and specializing in machine learning, I was curious to see if the two could be combined. I wrote a short proposal containing three possible ways to combine the two fields, and sent it off to potential supervisors.
Together with my supervisor (Pieter Adriaans, who would later also become my promotor), I landed on the topic of fractals to be combined with machine learning. I approached the topic from various angles, each of which became a chapter. Back then, we could still write thesis of over a hundred pages.
None of the results were truly convincing by themselves, but taken together, I think there is hint there of something that we still haven’t quite comprehended, even in the age of machine learning. One idea that I didn’t have time to work out in my master’s ultimately became a chapter of my PhD thesis: the use of the EM algorithm in fitting Iterated Function Systems to data.
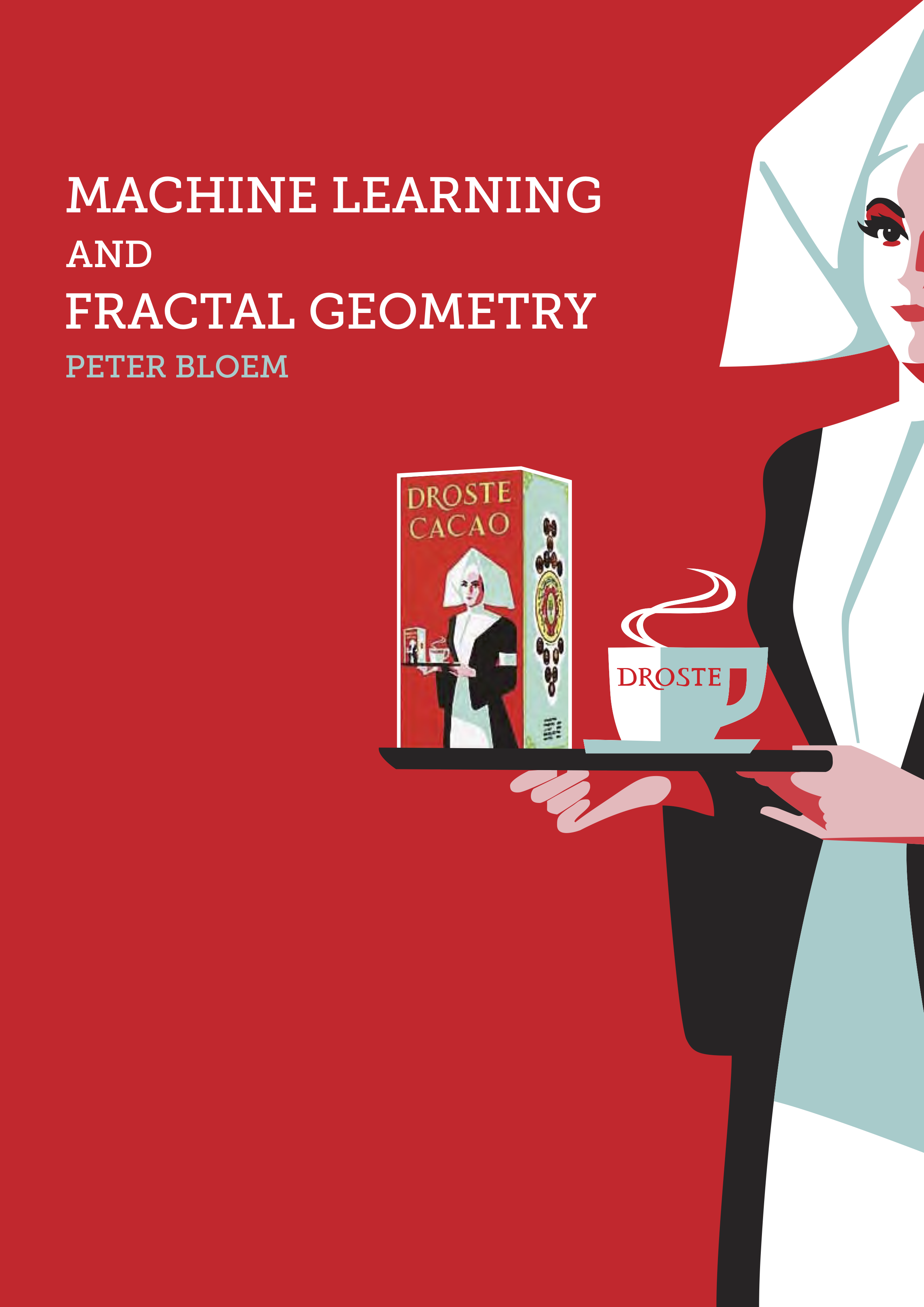